The Hidden Energy Cost Behind AI's Rise
When you think about artificial intelligence, you might picture sleek data centers with blinking lights or the convenience of asking Siri about tomorrow’s weather. What you probably don’t consider is the massive amount of electricity powering these technologies. As AI continues its explosive growth across industries, its energy consumption is skyrocketing – creating both challenges and opportunities for businesses and our planet.
We’re tracking this trend closely because it impacts nearly every sector we advise. The intersection of AI and electricity demand represents one of the most significant shifts in energy consumption patterns we’ve seen in decades.
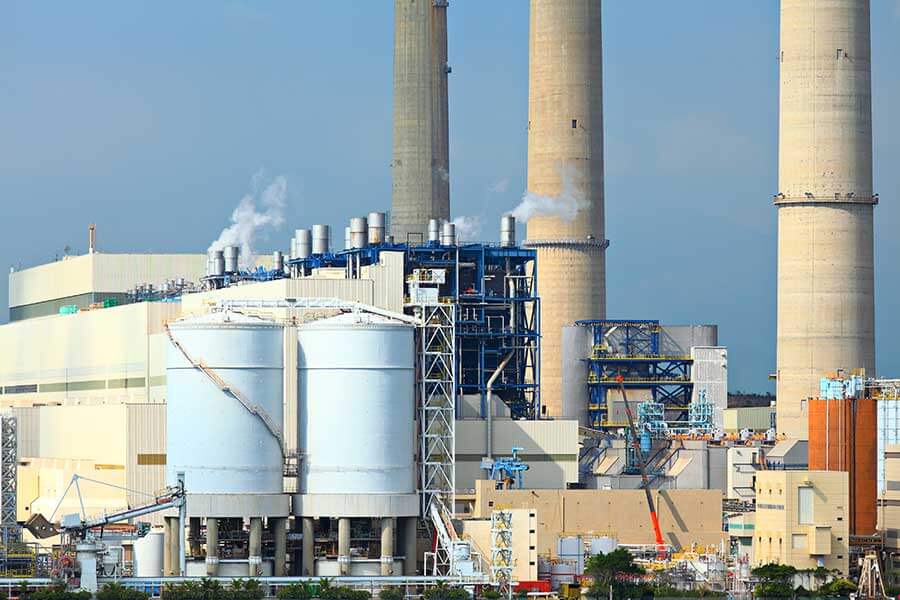
AI's Energy Footprint: Bigger Than You Think
The numbers are startling. Training a single advanced AI model can consume as much electricity as 100 U.S. households use in an entire year. And that’s just the beginning.
Data Centers: The Power-Hungry Brains Behind AI
24/7 Operation: The Always-On Nature of AI
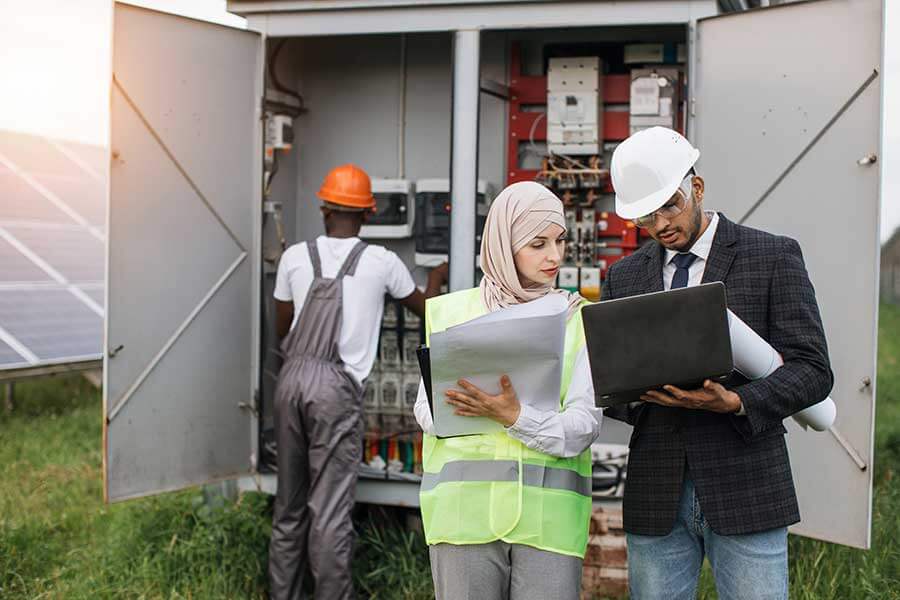
Global Impact: How AI is Reshaping Electricity Markets
Geographic Concentration
Timing Challenges
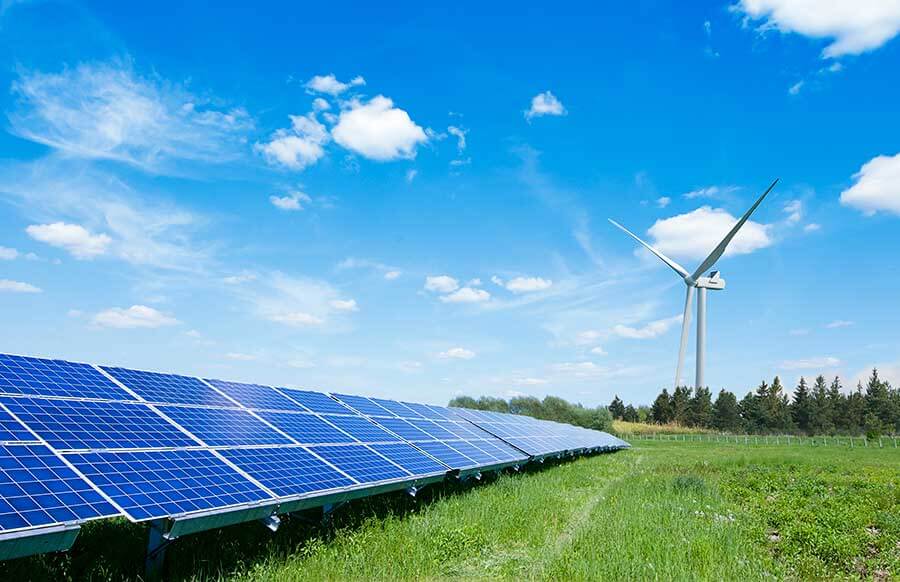
The Sustainability Question: Can AI and Green Energy Coexist?
The Carbon Footprint of AI
AI as Part of the Solution
Interestingly, AI itself may help address some of the energy challenges it creates. Machine learning systems are increasingly used to:
- Optimize power grid operations
- Predict renewable energy production
- Reduce energy waste in buildings and industrial processes
- Design more energy-efficient computer chips
We’ve seen firsthand how AI-powered energy management can reduce electricity consumption by 15-30% in commercial buildings.
What This Means for Your Business
Direct Costs
Strategic Planning
For forward-thinking businesses, understanding the connection between AI and electricity demand opens strategic opportunities:
- Energy-efficient AI implementations can provide competitive advantages
- Location decisions for new facilities should consider local electricity costs and grid capacity
- Sustainability commitments may need to account for AI’s energy use
Industry-Specific Impacts
Manufacturing
Healthcare
Financial Services
Looking Ahead: The Future of AI and Electricity Demand
Technological Advancements
Recent research shows promising developments in more energy-efficient AI architectures. Techniques like quantization, pruning, and specialized hardware design could significantly reduce electricity requirements for certain applications.
Policy and Regulation
Governments worldwide are beginning to acknowledge the energy implications of widespread AI adoption. New regulations may soon require energy disclosures for AI systems or incentivize more efficient designs.
Market Forces
As electricity costs become a larger component of AI expenses, market pressures will naturally drive innovation toward energy efficiency. Companies that solve the electricity demand challenge will gain significant competitive advantages.
Taking Action Now
While the relationship between AI and electricity demand presents challenges, proactive businesses can turn these challenges into opportunities:
- Start measuring the energy consumption of your AI systems
- Include electricity costs in your AI investment calculations
- Consider both the direct and indirect electricity demands when selecting AI vendors
- Explore how AI can help reduce your organization’s overall energy use
By addressing these issues now, you’ll be better positioned as AI continues to transform the business landscape.
Frequently Asked Questions About AI Energy Consumption
Training a large AI model can consume more electricity than many other computing tasks. For example, training a state-of-the-art natural language processing model might use as much electricity as 5-10 traditional software applications running for a full year.
While individual AI models are becoming more efficient, the overall electricity demand continues to grow as AI applications multiply and become more complex. The net effect is increasing total consumption despite efficiency improvements.
Successfully balancing AI implementation with sustainability requires a strategic approach that includes measuring energy impact, choosing efficient AI architectures, powering systems with renewable energy when possible, and using AI itself to optimize overall energy use.
Cloud providers typically operate more efficiently than individual company data centers. However, the convenience of cloud-based AI can also lead to increased usage and, consequently, more electricity consumption overall. The key is thoughtful implementation rather than simply shifting where the electricity is used.
Energy costs may appear directly in data center utility bills or be bundled into cloud service fees. Either way, they represent a growing portion of the total cost of ownership for AI systems.